Provide robust integrated functionality including a highly scalable database for all project data including a data catalog, and a file manager.
Multimodal Data Platforms:
A 2025 Buyer’s Guide
Evaluation criteria to select the right platform to meet biopharma’s biggest objectives
Get your copy
Drug discovery is expensive and inefficient
$2.9B
the cost1 of bringing a new drug to market
10-15 years
is the typical drug development timeframes2 with candidate nomination, selection, and approval comprising 21-26 months3
17%
of druggable proteins4 are targeted by U.S. FDA approved drugs
1. “New Research: Big Pharma Companies Earn Big Revenues Through Patent Gaming,” press release, America’s Health Insurance Plans, December 13, 2021, https://t.ly/rwksc
2. “Drug development – The four phases,” article, Biostock, January 2, 2023, https://t.ly/3kKbq
3. Gaurav Agrawal, Felix Bader, Jan Günthner, & Stephan Wurzer, “Fast to first-in-human: Getting new medicines to patients more quickly,” article, McKinsey, February 10, 2023, https://t.ly/L-QcD
4. "The Druggable Proteome", bit.ly/4fXcMKD
Multimodal data is driving innovation
Multimodal data, which critically includes large-scale high dimensional omics data, is the next frontier where biopharma is hunting for new targets to expand treatment capabilities and improve patient outcomes.
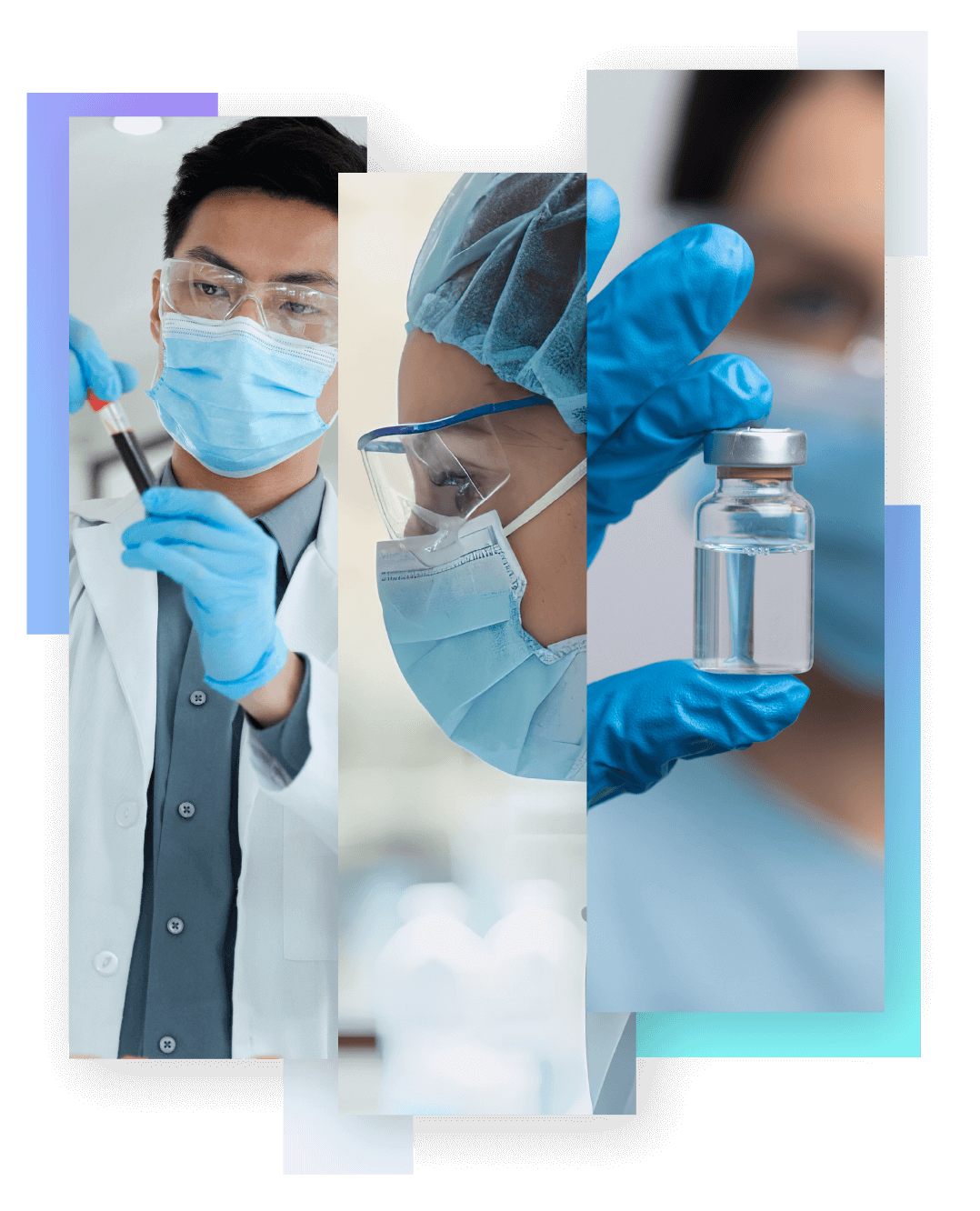
"We now have the practicality of doing multiomics at scale where you have sufficient statistical power to make any kind of useful conclusions. But we're only just starting to get there—the data is complex."
Head of Research IT
Global Pharma Company
"Multiomics is indispensable if you want to stay ahead of the curve. If everyone else has better tools than you to discover targets, they will generate better products.”
Senior Scientist
Global Biotechnology Company
Key buyers with shared interests



.png?width=50&height=219&name=image%20(20).png)
- Accelerate data processes
- Streamline cross-functional collaboration
- Speed time to insights
9 criteria to look for in a Multimodal R&D Platform
01. Offer a scientific system of record
02. Offer a unified data model
Support sparse and dense multi-dimensional arrays and capture different types of multiomics data.
03. Enable vector search
Support vector search and generative AI applications.
04. Abide by FAIR data principles
Ensure data and metadata is well-organized, so it is findable, accessible, interoperable, and reusable.
05. Support emerging data types
Provide a list of currently supported data and share plans for new data types and omics methodologies to be added.
06. Provide computational firepower
Deliver scalable computational power (e.g., CPUs, GPUs) and may leverage high-performance computing (HPC) environments.
07. Facilitate unsupervised learning
Deliver ML-ready data and enable teams to train models on vast datasets (such as tens of millions of single cells).
08. Ensure regulatory compliance
Comply with GDPR, HIPAA, ISO 27001, and SCDM regulations and SOC 2 controls.
09. Provide strong access controls
Provide project, group, and user settings, with role-based permissions on who can view, edit, and process data, as well as audit trails.